BaringhausHenzeTest
BaringhausHenzeTest[data]
Baringhaus–Henze検定を使い,データがMultinormalDistributionに従っているかどうかの検定を行う.
BaringhausHenzeTest[data,MultinormalDistribution[μ,Σ]]
データが,平均ベクトル μ,共分散行列 Σ で分布に従っているかどうかの検定を行う.
BaringhausHenzeTest[data,"property"]
"property"の値を返す.
詳細とオプション
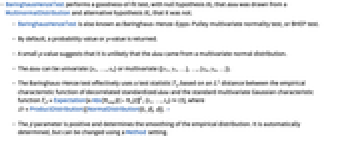
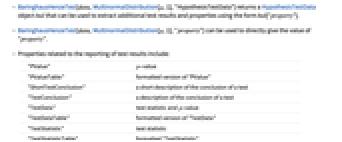
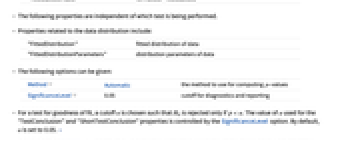
- BaringhausHenzeTestは,data がMultinormalDistributionから取られたという帰無仮説
とそうではないという対立仮説
で適合度検定を実行する.
- BaringhausHenzeTestは,Baringhaus–Henze–Epps–Pulley多変量正規性検定,つまりBHEP検定としても知られている.
- デフォルトで,確率値すなわち
値が返される.
- 小さい
値は data が多変量正規分布から来ている可能性が低いことを示す.
- data は,一変量{x1,…,xn}あるいは多変量{{x1,y1,…},…,{xn,yn,…}}でよい.
- Baringhaus–Henze検定は,事実上,無相関化された標準化 data と標準多変量ガウス特性関数Tβ=Expectation[n Abs[Ψemp[t]-Ψst[t]]2,{t1,…,td}]の間の距離
に基づく経験特性関数検定統計 Tβを使う.ただし,=ProductDistribution[{NormalDistribution[0,β],d}]である. »
- β 母数は正で,経験分布の平滑化を決定する.これは自動的に決定されるが,Method設定で変えることもできる.
- BaringhausHenzeTest[data,MultinormalDistribution[μ,Σ],"HypothesisTestData"]はHypothesisTestDataオブジェクト htd を返す.これは,htd["property"]として追加的な検定結果および特性の抽出に使うことができる.
- BaringhausHenzeTest[data,MultinormalDistribution[μ,Σ],"property"]を使って直接"property"の値を与えることができる.
- 検定結果のレポートに関連する特性
-
"PValue" 値
"PValueTable" "PValue"のフォーマットされたバージョン "ShortTestConclusion" 検定結果の簡単な説明 "TestConclusion" 検定結果の説明 "TestData" 検定統計と 値
"TestDataTable" "TestData"のフォーマットされたバージョン "TestStatistic" 検定統計 "TestStatisticTable" フォーマットされた"TestStatistic" - 次の特性はどの検定が行われているかに依存しない.
- データ分布に関連する特性
-
"FittedDistribution" データのフィットした分布 "FittedDistributionParameters" データの分布母数 - 使用可能なオプション
-
Method Automatic 値を計算するメソッド
SignificanceLevel 0.05 診断とレポートのための切捨て - 適合度検定では,
のときにのみ
が棄却されるような切捨て
が選択される.特性"TestConclusion"および"ShortTestConclusion"で使われる
の値はSignificanceLevelオプションで制御される.デフォルトで,
は0.05に設定されている. »
- Method->"MonteCarlo"の設定では,入力
と同じ長さのデータ集合が,フィットされた分布を使って
のもとで生成される.次に,BaringhausHenzeTest[si,"TestStatistic"]からのEmpiricalDistributionを使って
値が推定される. »
- Method{method,"SmoothingParameter"β}と設定すると,カスタムの平滑化母数 β を使うことができる.デフォルトは
である.この場合,検定はHenze-Zirkler検定としても知られるものになる. »
例題
すべて開くすべて閉じるスコープ (6)
検定 (3)
一変量の正規性についてBaringhaus–Henze検定を行う:
多変量の正規性についてBaringhaus–Henze検定を行う:
関連特性の抽出のためにHypothesisTestDataオブジェクトを作る:
オプション (3)
アプリケーション (4)
Baringhaus–Henze検定の検出力曲線(が偽であるときにこれを拒絶する確率):
基礎となる分布がMultivariateTDistributionであり,検定サイズが0.05,サンプルサイズが43である場合の,Baringhaus–Henze検定の検出力を推定する:
連続時間ランダム過程がガウス過程であるかどうかを調べる.非整数ブラウン運動:
コックス・インガソール・ロス(Cox–Ingersoll–Ross)過程の対数の差:
治療研究の前と後の患者の体重が記録されている.データが多変量正規分布に従っていれば,平均の多変量検定を使って対照群と実験群を識別することができる:
BaringhausHenzeTestを使って,データの3群が多変量正規分布に従っているかどうかを判定する:
Baringhaus–Henze検定はContおよびFTの群を棄却しなかった.TTestを使ってこの2群の平均が等しいかどうかを見る:
特性と関係 (5)
Baringhaus–Henze検定は,データに適用された線形変換が非特異であればアフィン不変である:
正規性についてのMardiaの結合検定はもまた,同じ特性を有する:
のもとでは,検定統計はLogNormalDistributionに漸近的に従う:
検定統計がLogNormalDistribution族からの分布に従うかどうかの検定を行う:
BaringhausHenzeTest統計は,帰無仮説のもとでサンプルの経験特性関数と特性関数の間の距離に基づいている:
BaringhausHenzeTestでレポートされた検定特性の値と比較する:
退化したサンプル共分散関数のサンプルについては,検定統計その最大値である4 n を与える:
テキスト
Wolfram Research (2015), BaringhausHenzeTest, Wolfram言語関数, https://reference.wolfram.com/language/ref/BaringhausHenzeTest.html.
CMS
Wolfram Language. 2015. "BaringhausHenzeTest." Wolfram Language & System Documentation Center. Wolfram Research. https://reference.wolfram.com/language/ref/BaringhausHenzeTest.html.
APA
Wolfram Language. (2015). BaringhausHenzeTest. Wolfram Language & System Documentation Center. Retrieved from https://reference.wolfram.com/language/ref/BaringhausHenzeTest.html