TransformAnomalies
✖
TransformAnomalies
transforms tabular data tab using the specification speci for column coli.
Details
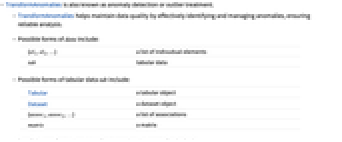
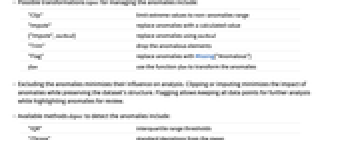
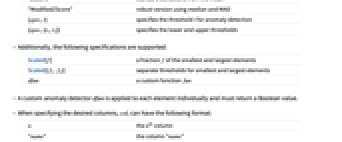
- TransformAnomalies is also known as anomaly detection or outlier treatment.
- TransformAnomalies helps maintain data quality by effectively identifying and managing anomalies, ensuring reliable analysis.
- Possible forms of data include:
-
{el1,el2,…} a list of indivudual elements tab tabular data - Possible forms of tabular data tab include:
-
Tabular a tabular object Dataset a dataset object {assoc1,assoc2,…} a list of associations matrix a matrix - Possible transformations tspec for managing the anomalies include:
-
"Clip" limit extreme values to non-anomalies range "Replace" replace anomalies with a calculated value {"Replace",method} replace anomalies using method "Trim" drop the anomalous elements "Flag" replace anomalies with Missing["Anomalous"] tfun use the function tfun to transform the anomalies - Possible "Replace" methods are the same as in TransformMissing:
-
" Mean" the mean value "Median" the median value "Mode" the most frequent value {"Constant", val} a constant value val "RandomElement" randomly selected value {"RandomVariate",dist} value sampled from dist "NearestElement" use the closest non-missing element "NextElement" use the next non-missing element "PreviousElement" use the previous non-missing element "Interpolation" use interpolation {"Interpolation",InterpolationOrderdegree} specify the interpolation order degree - Excluding the anomalies minimizes their influence on analysis. Clipping or replacing minimizes the impact of anomalies while preserving the dataset's structure. Flagging allows keeping all data points for further analysis while highlighting anomalies for review.
- Available methods dspec to detect the anomalies include:
-
"IQR" interquartile range thresholds "ZScore" standard deviations from the mean "ModifiedZScore" robust version using median and MAD {spec,t} specifies the threshold t for anomaly detection {spec,{t1,t2}} specifies the lower and upper thresholds - Additionally, the following specifications are supported:
-
Scaled[f] a fraction f of the smallest and largest elements Scaled[{f1,f2}] separate thresholds for smallest and largest elements dfun a custom function fun - A custom anomaly detector dfun is applied to each element individually and must return a Boolean value.
- When specifying the desired columns, coli can have the following format:
-
n the n column
"name" the column "name" TypeSpecifier[…] columns matching the specified type All all the columns Automatic automatically find columns based on spec (default)
Examples
open allclose allBasic Examples (2)Summary of the most common use cases
Scope (16)Survey of the scope of standard use cases
Data Types (5)

https://wolfram.com/xid/0bzqjb04t4ot63dhw-5qw5ki

Detect anomalies in a TabularColumn:

https://wolfram.com/xid/0bzqjb04t4ot63dhw-3skyhj
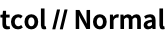
https://wolfram.com/xid/0bzqjb04t4ot63dhw-wo232c
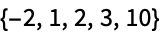

https://wolfram.com/xid/0bzqjb04t4ot63dhw-8kwc1q

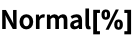
https://wolfram.com/xid/0bzqjb04t4ot63dhw-zc874m

Detect anomalies along matrix columns:

https://wolfram.com/xid/0bzqjb04t4ot63dhw-i31bl4

Detect anomalies in a Tabular object:
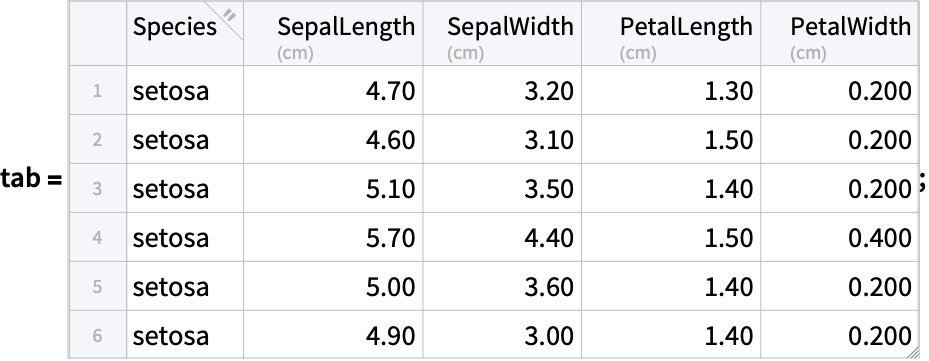
https://wolfram.com/xid/0bzqjb04t4ot63dhw-4u2u8i

https://wolfram.com/xid/0bzqjb04t4ot63dhw-xq3pwz
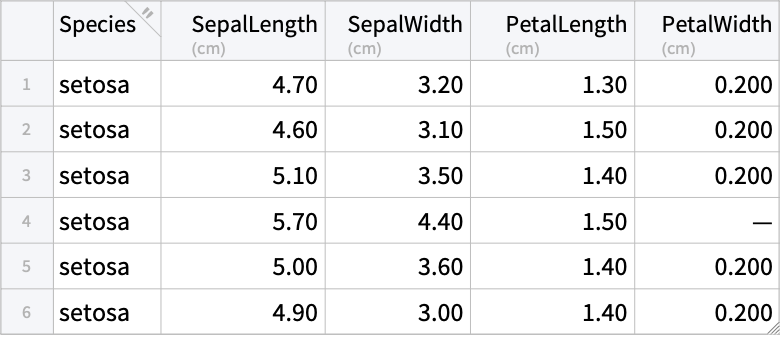
Detect anomalies in a list of associations across the same key:

https://wolfram.com/xid/0bzqjb04t4ot63dhw-hxlxl6

Detection Methods (6)
Detect anomalies using the interquartile range:

https://wolfram.com/xid/0bzqjb04t4ot63dhw-1wnbug

Detect anomalies using the z-score:

https://wolfram.com/xid/0bzqjb04t4ot63dhw-cx9jx4
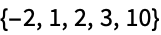
Detect anomalies using the modified z-score:

https://wolfram.com/xid/0bzqjb04t4ot63dhw-6ws6qo


https://wolfram.com/xid/0bzqjb04t4ot63dhw-cmyee3

Specify upper and lower thresholds individually:

https://wolfram.com/xid/0bzqjb04t4ot63dhw-q63xa2

Flag a fraction of the smallest and largest elements:

https://wolfram.com/xid/0bzqjb04t4ot63dhw-zm5drg

Specify separate smallest and largest fractions:

https://wolfram.com/xid/0bzqjb04t4ot63dhw-0oi5in

Use a custom function to detect anomalies:

https://wolfram.com/xid/0bzqjb04t4ot63dhw-ezv21d

Transformation Methods (5)

https://wolfram.com/xid/0bzqjb04t4ot63dhw-lwf4ma
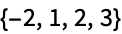
Clip the anomalies with the closest valid value:

https://wolfram.com/xid/0bzqjb04t4ot63dhw-y8sj87
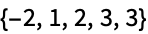
Replace the anomalies with missing elements:

https://wolfram.com/xid/0bzqjb04t4ot63dhw-74aib9

Apply any TransformMissing specification:

https://wolfram.com/xid/0bzqjb04t4ot63dhw-vvx7e5
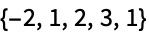
Apply a custom transformation:

https://wolfram.com/xid/0bzqjb04t4ot63dhw-fb9oxh

Applications (2)Sample problems that can be solved with this function
Take a Tabular object of sales data:
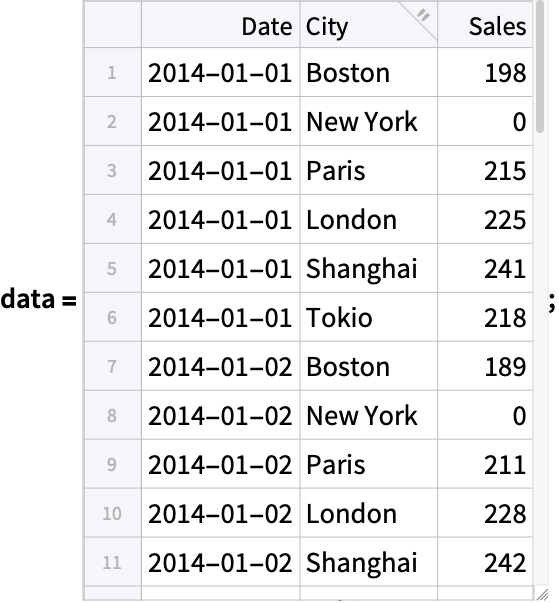
https://wolfram.com/xid/0bzqjb04t4ot63dhw-h39wd1
The "Sales" column contains outlier zero values, which are standing in for missing values:

https://wolfram.com/xid/0bzqjb04t4ot63dhw-cre4sv
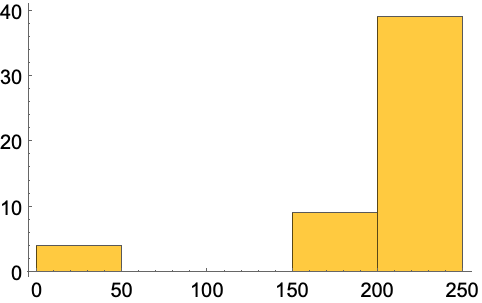
Transform the zero anomalies by removing the relevant rows from the original data:

https://wolfram.com/xid/0bzqjb04t4ot63dhw-y3xwc
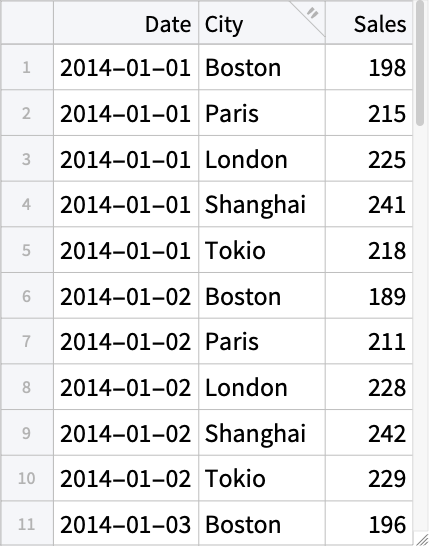
Transform the zero anomalies by converting them to Missing objects:

https://wolfram.com/xid/0bzqjb04t4ot63dhw-itutb4
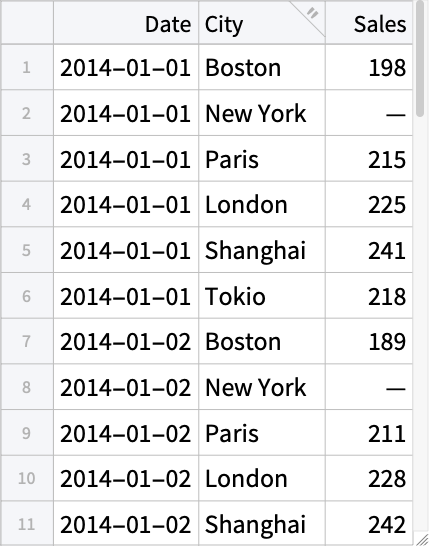

https://wolfram.com/xid/0bzqjb04t4ot63dhw-qmp448
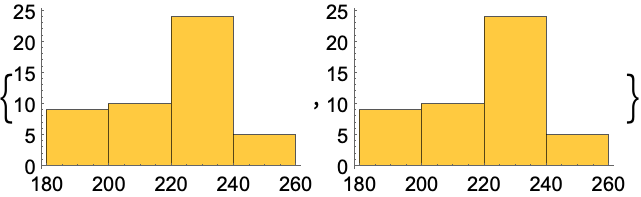
Take a Tabular object of weather observations:
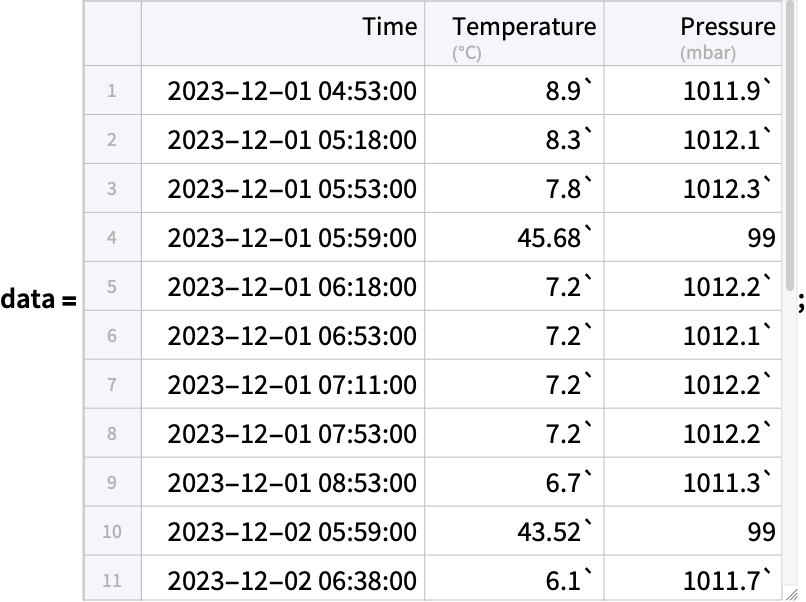
https://wolfram.com/xid/0bzqjb04t4ot63dhw-fha1be

https://wolfram.com/xid/0bzqjb04t4ot63dhw-2hkg3k
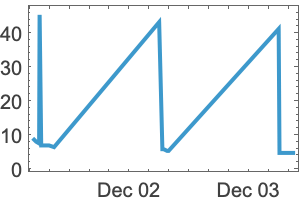
It appears the temperature data contains outliers, possibly resulting from entering the data in wrong units. Similarly, the "Pressure" column contains outliers, that is number 99, which is often used in place of missing values. Specify the temperature unit conversion function:

https://wolfram.com/xid/0bzqjb04t4ot63dhw-mks3kb
Transform anomalies using the above function for the "Temperature" column and in the "Pressure" column replace the anomaly with the nearest valid value:

https://wolfram.com/xid/0bzqjb04t4ot63dhw-rtj7di
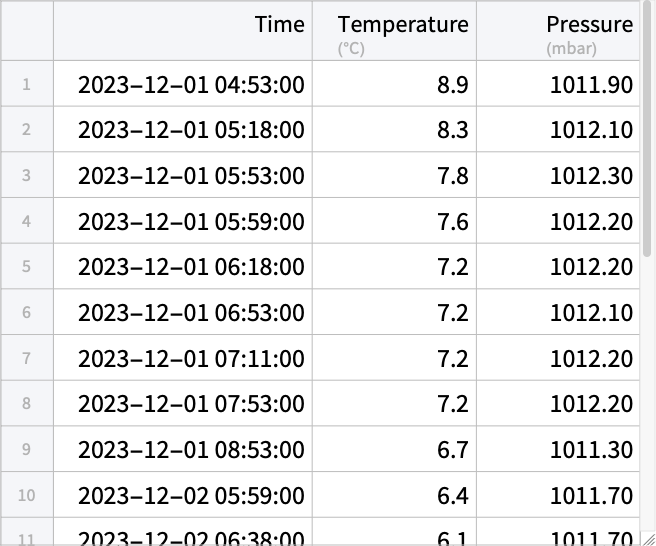

https://wolfram.com/xid/0bzqjb04t4ot63dhw-wnfy3e
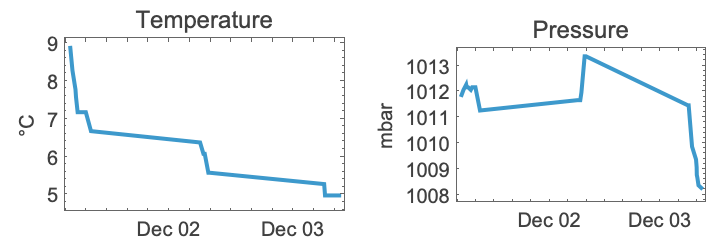
Wolfram Research (2025), TransformAnomalies, Wolfram Language function, https://reference.wolfram.com/language/ref/TransformAnomalies.html.
Text
Wolfram Research (2025), TransformAnomalies, Wolfram Language function, https://reference.wolfram.com/language/ref/TransformAnomalies.html.
Wolfram Research (2025), TransformAnomalies, Wolfram Language function, https://reference.wolfram.com/language/ref/TransformAnomalies.html.
CMS
Wolfram Language. 2025. "TransformAnomalies." Wolfram Language & System Documentation Center. Wolfram Research. https://reference.wolfram.com/language/ref/TransformAnomalies.html.
Wolfram Language. 2025. "TransformAnomalies." Wolfram Language & System Documentation Center. Wolfram Research. https://reference.wolfram.com/language/ref/TransformAnomalies.html.
APA
Wolfram Language. (2025). TransformAnomalies. Wolfram Language & System Documentation Center. Retrieved from https://reference.wolfram.com/language/ref/TransformAnomalies.html
Wolfram Language. (2025). TransformAnomalies. Wolfram Language & System Documentation Center. Retrieved from https://reference.wolfram.com/language/ref/TransformAnomalies.html
BibTeX
@misc{reference.wolfram_2025_transformanomalies, author="Wolfram Research", title="{TransformAnomalies}", year="2025", howpublished="\url{https://reference.wolfram.com/language/ref/TransformAnomalies.html}", note=[Accessed: 13-May-2025
]}
BibLaTeX
@online{reference.wolfram_2025_transformanomalies, organization={Wolfram Research}, title={TransformAnomalies}, year={2025}, url={https://reference.wolfram.com/language/ref/TransformAnomalies.html}, note=[Accessed: 13-May-2025
]}