PointCountDistribution
PointCountDistribution[pproc,reg]
represents the distribution of point counts for the point process pproc in the region reg.
PointCountDistribution[pproc,{reg1,…,regn}]
represents the joint distribution of point counts in regions regi.
Details
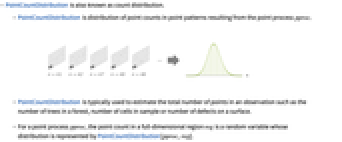
- PointCountDistribution is also known as count distribution.
- PointCountDistribution is distribution of point counts in point patterns resulting from the point process pproc.
- PointCountDistribution is typically used to estimate the total number of points in an observation such as the number of trees in a forest, number of cells in sample or number of defects on a surface.
- For a point process pproc, the point count in a full-dimensional region reg is a random variable whose distribution is represented by PointCountDistribution[pproc,reg].
- The mean of the PointCountDistribution[pproc,reg] is the mean measure of the point process pproc in the region reg.
- The regions regi and reg should be full dimensional and bounded as tested by SpatialObservationRegionQ, but in special cases can be defined with parameters.
- PointCountDistribution will simplify to known special distributions whenever possible.
- PointCountDistribution can be used with such functions as Mean, CDF and RandomVariate, etc.
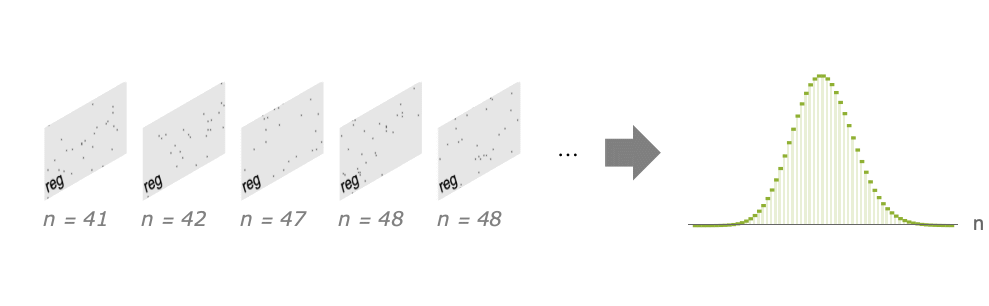
Examples
open allclose allBasic Examples (3)
Distribution of the number of points for a PoissonPointProcess in a unit disk:
Find mean and variance of the number of points:
PointCountDistribution for a HardcorePointProcess in a unit ball:
PointCountDistribution for a GeoDisk:
Scope (4)
PointCountDistribution of a BinomialPointProcess on a unit disk:
The distribution of the number of points in a subregion:
Compute the mean number of points:
PointCountDistribution of a InhomogeneousPoissonPointProcess over a unit disk:
Over the union of two disjoint regions:
Over two intersecting regions:
PointCountDistribution for cluster processes of Neyman–Scott type is a CompoundPoissonDistribution:
In general, the point count distribution of a Neyman–Scott point process does not depend on the spatial distribution of the cluster points:
Compute probabilities and expectations in the absence of the closed form of PointCountDistribution:
Applications (5)
Compute the average number of points in a unit disk for a Strauss point process:
Suppose flaws in plywood occur on an average of one flaw per 50 square feet. Simulate the process of finding flaws on a per-square-foot basis:
The number of flaws in a given region:
Find the probability that a 4-foot-by-8-foot sheet will have no flaws:
For a round mirror with area 7.54 cm, the probability of no flaws is 0.91. Using the same polishing process, another round mirror with an area of 19.50 cm
is fabricated. Assuming the flaws are independent and randomly located, find the probability of no flaws on the larger mirror:
The distribution of the number of flaws in the mirror:
Find the intensity of the flaw point process:
The distribution of the number of flaws in the second mirror:
The probability of no errors in the larger mirror:
An LCD display has 1920×1080 pixels. A display is accepted if it has 15 or fewer faulty pixels. The probability that a pixel is faulty from production is and the faulty pixel positions are independent and random. Find the proportion of displays that are accepted:
Simulate the faulty pixel configuration:
The distribution of the number of faulty pixels in the display:
Find the probability of no more than 15 faulty pixels in the display:
Find the pixel failure rate required to produce 4000×2000 pixel displays and still have an acceptance rate of at least 90%:
Plot the acceptance rate as a function of the pixel failure rate:
Find the maximal acceptable pixel failure rate:

Incidents of car-related thefts in Chicago:
Define inhomogeneous Poisson point process with this intensity:

Find the mean number of crimes:
Compute the probability that the number of crimes is less than 1200:
Compute the expected number of incidents in ZIP code 60639:

Properties & Relations (4)
PointCountDistribution over pairwise disjoint region is a ProductDistribution:
PointCountDistribution over each region:
Multivariate PointCountDistribution over the list of regions:
PointCountDistribution over overlapping regions:
PointCountDistribution over each region:
Multivariate PointCountDistribution over the list of regions:
The components are correlated where the regions intersect:
PointCountDistribution over region covering for BinomialPointProcess:
Point count distribution for the covering:
PointCountDistribution for a region with non-numeric parameters:
Text
Wolfram Research (2020), PointCountDistribution, Wolfram Language function, https://reference.wolfram.com/language/ref/PointCountDistribution.html.
CMS
Wolfram Language. 2020. "PointCountDistribution." Wolfram Language & System Documentation Center. Wolfram Research. https://reference.wolfram.com/language/ref/PointCountDistribution.html.
APA
Wolfram Language. (2020). PointCountDistribution. Wolfram Language & System Documentation Center. Retrieved from https://reference.wolfram.com/language/ref/PointCountDistribution.html